A nonlinear method for monitoring industrial process
Aiming at fault detection in industrial processes with nonlinear or high dimensions, a novel method based on locally linear embedding preserve neighborhood for fault detection is proposed in this paper. Locally linear embedding preserve neighborhood is a feature-mapping method that combines Locally linear embedding and Laplacian eigenmaps algorithms. First, two weight matrices are obtained by the Locally linear embedding and Laplacian eigenmaps, respectively. Subsequently, the two weight matrices are combined by a balance factor to obtain the objective function. Locally linear embedding preserve neighborhood method can effectively maintain the characteristics of data in high-dimensional space. The purpose of dimension reduction is to map the high-dimensional data to low-dimensional space by optimizing the objective function. Process monitoring is performed by constructing T2 and Q statistics. To demonstrate its effectiveness and superiority, the proposed locally linear embedding preserve neighborhood for fault detection method is tested under the Swiss Roll dataset and an industrial case study. Compared with traditional fault detection methods, the proposed method in this paper effectively improves the detection rate and reduces the false alarm rate.
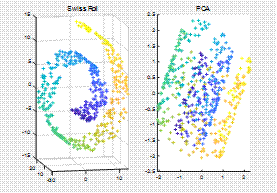
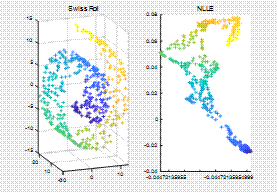
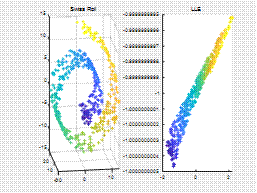
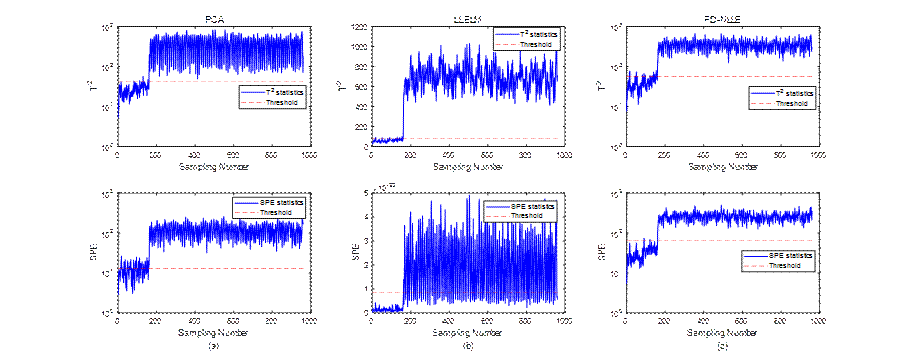