A Novel Fault Detection Scheme Based on Difference in Independent Component for Reliable Process Monitoring: Application on the Semiconductor Manufacturing Processes
Compared with principal component analysis (PCA)-based methodologies, independent component analysis (ICA) is more suitable for extracting the variation information of latent variables. The advanced fault detection approach based on ICA utilizes two statistics, I2d and square prediction error (SPE), to monitor the health status of a single mode process. However, when these statistics are used for monitoring a multimode process, their fault detection performances are disappointed.
Aiming to detect some faults caused by abnormal changes of latent variables in a multimode process, a novel health status monitoring scheme based on dierence in independent component (Diff-ICA) is proposed in this paper. InDiff-ICA, the conventional ICA is firstly implemented to extract the latent information of a process. Next, the estimated independent components of each sample are calculated using k nearest neighbors (kNN) rule. After that, a new statistic I2diff, which is built based on the difference between real independent components and estimated independent components,is used for monitoring the variation of latent variables in this process. Meanwhile, another statistic qdiff, which isbuilt in the residual subspace that is reconstructed using these estimated independent components, is applied to monitor the health status of this process. Diff-ICA is capable of both reducing the impact of multimode characteristics on process monitoring and improving fault detection rate of a multimode process. The efficiency of Diff-ICA is demonstrated by a simulated case as well as an industrial case. The experimental results indicate that the proposed method outperforms PCA, kernel PCA (KPCA), ICA and FD-kNN.
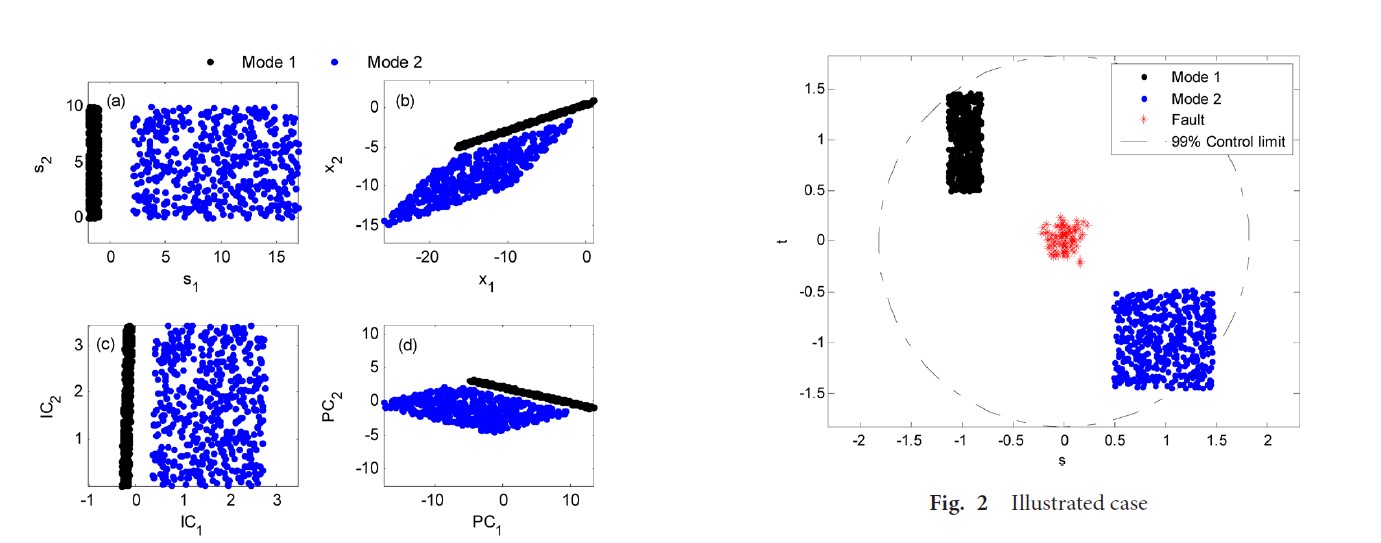